Noise suppression and active noise reduction: what are the differences
Time:2022-10-14
Views:1689
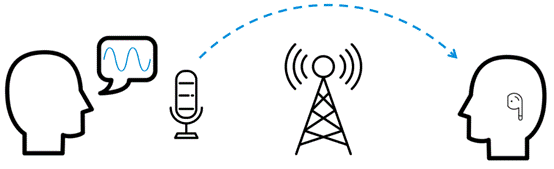
This is due to noise suppression and active noise reduction (ANC). These two functions are very common in audio products recently, but they are not just buzzwords. These two technologies help mitigate the effects of noise in different important ways. This paper will explain the difference between the two, and discuss the noise suppression technology more deeply.
noise suppression
Look at the first part of the above scenario: you speak into the microphone in a noisy environment.
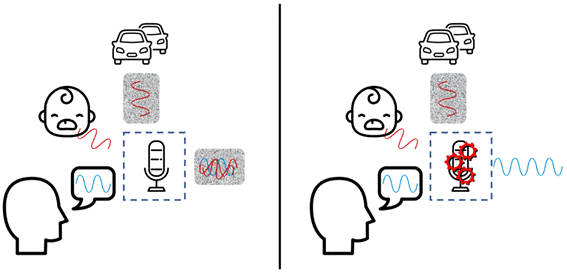
In this example, the car is emitting continuous background noise. This is the so-called steady state noise, which shows the periodic characteristics that do not exist in the speech signal we are concerned about. The sound of air conditioners, aircraft, car engines and fans are examples of steady state noise. However, the crying of infants is not continuous, and this sound has a very appropriate name: non-stationary noise. Other examples of such noise include the barking of a husky, the sound of an electric drill or hammer working, the click of a keyboard or the clink of silverware in a restaurant. These noises occur suddenly and exist for a short time at the same time.
The microphone captures both types of noise; Without any processing, it will produce the same noisy output, which will cover the voice information you expect to transmit. The left half of the figure shows this. However, with noise suppression processing, you can eliminate background noise in order to transmit (and only transmit) your voice.
Active noise reduction
Now, your clear voice has been transmitted to your friend by radio, but this does not mean that the other party can receive your message clearly. At this time, active noise reduction is required.
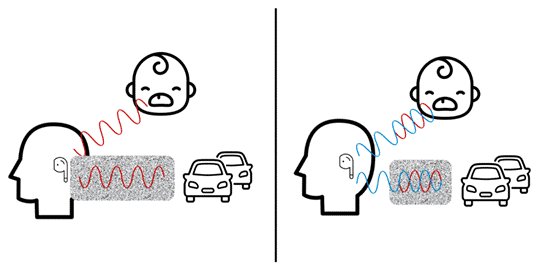
As before, steady and unsteady noise can affect the sound coming into the earplug. The objective of active noise reduction is to completely eliminate the external noise, which is different from the previous noise elimination. The microphone captures the incoming sound, generates the reverse signal of the external noise, and cancels the external noise as much as possible by superimposing the reverse signal into the sound wave in the ear. To summarize, this is conceptually similar to adding two numbers,+5 and - 5, to get 0.
In hardware, based on the above basic principles, active noise reduction can be applied in two main ways. One is the feedforward ANC, that is, the microphone is used outside the audible device; The other is feedback ANC, which uses a microphone in an audible device closer to the ear.
The feedforward ANC is located outside the ear, so it is more sensitive to noise. It can clearly capture noise as it travels to audible devices. It can then process the noise and output its phase cancellation signal. This allows it to isolate specific sounds, especially intermediate frequencies. This includes the steady sound we mentioned earlier in this post, but also includes voice. However, the feed-forward ANC is located outside the device, so it is more vulnerable to external noise, such as wind noise or the sound of earplugs rubbing on the inside of the hood (this is definitely not empirical).
The feedback ANC is not affected by the moving hood, because it is inside the audible device and can resist other kinds of accidental interference. This sound insulation is very good, but the higher frequency sound that is successfully introduced into the earplug is more difficult to offset. Similarly, the internal feedback microphone needs to distinguish between the music playing and the noise. Also, because its feedback is closer to the ear, it needs to process this information faster to maintain the same delay as the feedforward setting.
Finally, there is hybrid active noise reduction. You guessed it. This method combines feedforward and feedback ANC to achieve the best results in both aspects at the cost of power consumption and hardware
Learn more about noise suppression
After understanding the basic difference between noise suppression (suppressing the speaker‘s environmental noise so that the remote listener can hear it clearly) and active noise reduction (canceling the listener‘s own environmental noise), let‘s focus on how to achieve noise suppression.
One method is to use multiple microphones to suppress data. Collect data from multiple locations, and the device will get similar (but still different) signals. The voice signal received by the microphone close to the speaker‘s department is obviously stronger than that of the secondary microphone. The two microphones will receive a non voice background tone of similar signal strength. Subtract the sound information collected by the strong voice microphone and the secondary microphone, and the rest is mostly voice information. The greater the distance between microphones, the greater the signal difference between the nearer and farther microphones, and the easier it is to use this simple algorithm to suppress noise. However, when you don‘t speak, or when the expected voice data changes over time (for example, when you walk or run, and the phone keeps shaking), the effect of this method will be reduced. Multi microphone noise suppression is certainly reliable, but additional hardware and processing have shortcomings.
What if there was only one microphone? If no additional sound source is used for verification/comparison, a single microphone solution will rely on understanding and filtering the received noise characteristics. This is related to the previously mentioned definitions of steady and unsteady noise. Steady state noise can be effectively filtered by DSP algorithm. Unsteady state noise brings a challenge, but deep neural network (DNN) can help solve the problem.
This method requires a data set for training the network. The data set is composed of different (steady and non-stationary) noises and clear speech to create a synthetic noisy speech mode. The data set is fed to DNN as input, and clear voice is used as output. This will create a neural network model that will eliminate noise and output only clear speech.
Even with trained DNNs, there are still some challenges and indicators to consider. If you want to run in real time with low latency, you need a strong processing power or a small DNN. The more parameters in DNN, the slower it runs. Audio sampling rate has a similar effect on sound suppression. A higher sampling rate means that DNN needs to handle more parameters, but in turn, it will get a better output. In order to realize real-time noise suppression, narrowband voice communication is an ideal choice.
This processing is all intensive tasks. Cloud computing is very good at completing such tasks, but this method will significantly increase the delay. Considering that humans can reliably distinguish the delay of more than 108 milliseconds, the additional delay caused by cloud computing processing is obviously not an ideal result. However, running DNN at the edge requires some clever adjustments. CEVA is always committed to improving our voice and voice processing capabilities. This includes proven speech intelligibility and command recognition algorithms that provide clear communication and voice control even at the edge. Welcome to contact us and listen in person.
Disclaimer: This article is transferred from other platforms and does not represent the views and positions of this site. If there is any infringement or objection, please contact us to delete it. thank you! |